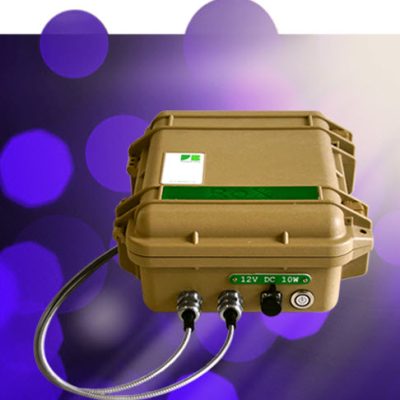
The reflectance box (ROX) is a robust tool for collecting hyperspectral time series of your environmental research area. Fully autonomous operation, a rugged weatherproof design paired with low power consumption make it a simple but sophisticated device for all kind of reflectance measurements.
The ROX is an automated field spectroscopy device capable of collecting unattended, continuous, long-term hyperspectral measurements. It was developed from a collaboration between Jülich Research Center and the Remote Sensing of Environmental Dynamics Laboratory of the University Milano Bicocca, and is designed for long term measurements of solar radiance, reflected radiance and reflectance. Its robustness and flexibility make the ROX suitable for numerous applications including vegetation monitoring (numerous reflective vegetation indices such as NDVI, PRI etc.), and monitoring water quality, snow and ice.
The opening angle of the downward looking fiber is 23°. The ROX may be mounted on a tower, or any convenient structure, at an appropriate height to capture data from the required area.
Typical tower set-ups for grass, corn and forest with reasonable size estimations based on working setups. Drawing not to scale
Data Processing
To generate reflectance, radiance and a long list of vegetation indices, an open source R-package is available at github. For simple use, the package is wrapped in a graphical user interface to process years of data with a few clicks.
Power
The ROX accepts 9-14 V via the main plug. But you can also power it directly via USB using your field notebook or a power bank for mobile phones.
Portability
The small case and the low power consumption make the ROX a perfect field spectrometer. Having an upward and another downward looking fiber also eliminates the need for a frequent white reference measurement. To trigger the instrument, you can use the USB connection or wireless communication. A fiber levelling gimbal ensure measurements are always nadir.
- Field measurements of Spectral Reflectance from Vegetation.
- Monitoring Plant Reflective Indices (NDVI; PRI; MCARI; SRPI etc.)
- Ground-truthing satellite data.
- Cryosphere studies
- Snow properties and melt prediction.
- Water quality of lakes and rivers
- Algal blooms
OPTICAL
- Wavelength range: VIS-NIR: ~ 400–950 nm (other options also available on demand)
- Spectral Sampling Interval (SSI): ~ 0.65 nm
- Spectral resolution (FWHM): ~ 1.5 nm
- Signal to Noise Ratio (SNR): ~ 250
- Field Of View (FOV): Upwelling radiance ~ 25°,Downwelling radiance 180°
OPERATIONAL
- Signal Optimization: Automatic adaption to varying light conditions
- Dark current: Accurate determination at each measurement cycle
- Manual acquisition: Interface software for manual measurement and calibration
- Automatic acquisition: Fully autonomous measurement mode for unattended data acquisition 20 seconds under bright sunshine 60 seconds in overcast conditions
- Quick measurements: 10 seconds under bright sunshine 30 seconds in overcast conditions
- Stability: Reference system stability check and uncertainty estimates
- Simultaneous metadata: Temperature, GPS position, GPS time
- Data storage: SD card up to 32 GB (12 months of measurements)
- Case: Robust and Waterproof housing based on the 1200 Pelicase
- Dimensions: 300 × 250 × 130 mm
- Power supply: 12 Volt. From battery and solar panels
- Power consumption: 800 mAh
- Energy saver: Day/night switch for energy saving
- Interfaces: RS232 via cable and wireless
OPTIONAL
- Dust Protection: Additional dust protection for Cosine Receptors
- Fiber optics: Flexible length of fiber optics according to user needs
- Power supply: Solar panel and battery
- Field use: Backpack option including small battery packs
A. Babic et al. (2023) Heterogenous Marine Robotic System for Environmental Monitoring Missions. 2023 IEEE Underwater Technology (UT), Tokyo, Japan, 2023, pp. 1-5, doi: 10.1109/UT49729.2023.10103383.
P. Naethe et al. (2023) Calibration and Validation from Ground to Airborne and Satellite Level: Joint Application of Time- Synchronous Field Spectroscopy, Drone, Aircraft and Sentinel-2 Imaging. PFG – Journal of Photogrammetry, remote Sensing and Geoinformation Science. https://doi.org/10.1007/s41064-022-00231-x
A Burkart et al. (2022) Iterative Design of a High Light Throughput Cosine Receptor Fore Optic for Unattended Proximal Remote Sensing. Jopurnal of Applied Remote Sensing Vol 16 (4) https://doi.org/10.1117/1.JRS.16.044513
E. Rommel et al. (2022) Very High-Resolution Imagery and Machine Learning for Detailed Mapping of Riparian Vegetation and Substrate Types. Remote Sensing 14(4), 954 https://doi.org/10.3390/rs14040954
I. Cesana et al. (2021) Preliminary Investigation on Phytoplankton Dynamics and Primary Production Models in an Oligotrophic Lake from Remote Sensing Measurements. Sensors 2021. https://www.mdpi.com/1424-8220/21/15/5072
A. Kokhanovsky, B. Di Mauro, R. Garzonio, R. Colombo (2021) Retrieval of Dust Properties From Spectral Snow Reflectance Measurements. Front. Environ. Sci. https://www.frontiersin.org/articles/10.3389/fenvs.2021.644551/full
P. M. Maier, S. Keller and S. Hinz (2021) Deep Learning with WASI Simulation Data for Estimating Chlorophyll a Concentration of Inland Water Bodies. Remotes sensing 2021. https://www.mdpi.com/2072-4292/13/4/718#cite
P. Näthe, M. Delaney, T. Julitta (2020) Changes of NOx in urban air detected with monitoring VIS-NIR field spectrometer during the coronavirus pandemic: A case study in Germany. Science of The Total Environment. https://www.sciencedirect.com/science/article/pii/S0048969720348154
A. Wagner S. Hilgert T. Kattenborn S. Fuchs (2019) Proximal VIS-NIR spectrometry to retrieve substance concentrations in surface waters using partial least squares modelling. Water Supply Vol 19. https://iwaponline.com/ws/article/19/4/1204/64503/Proximal-VIS-NIR-spectrometry-to-retrieve
B. Di Mauro et al. (2020) PRISMA hyperspectral satellite mission: first data on snow in the Alps, EGU General Assembly https://meetingorganizer.copernicus.org/EGU2020/EGU2020-19825.html
T. Julitta et al. (2018) Exploiting top of Canopy Sun Induced Chlorophyll Fluorescence by the FloX. From Instrument performance to data processing. OPTIMISE COST Action Final Meeting. 21-23 February 2018, Sofia (Bulgaria) https://optimise.dcs.aber.ac.uk/optimise-final-conference/
K. Biriukova et al. (2018) Characterisation of reflectance and chlorophyll fluorescence anisotropy – defining requirements for an experimental setup. Geophysical Research Abstracts, vol. 20, EGU2018-16936, 2018. EGU General Assembly 2018 https://meetingorganizer.copernicus.org/EGU2018/EGU2018-16936.pdf
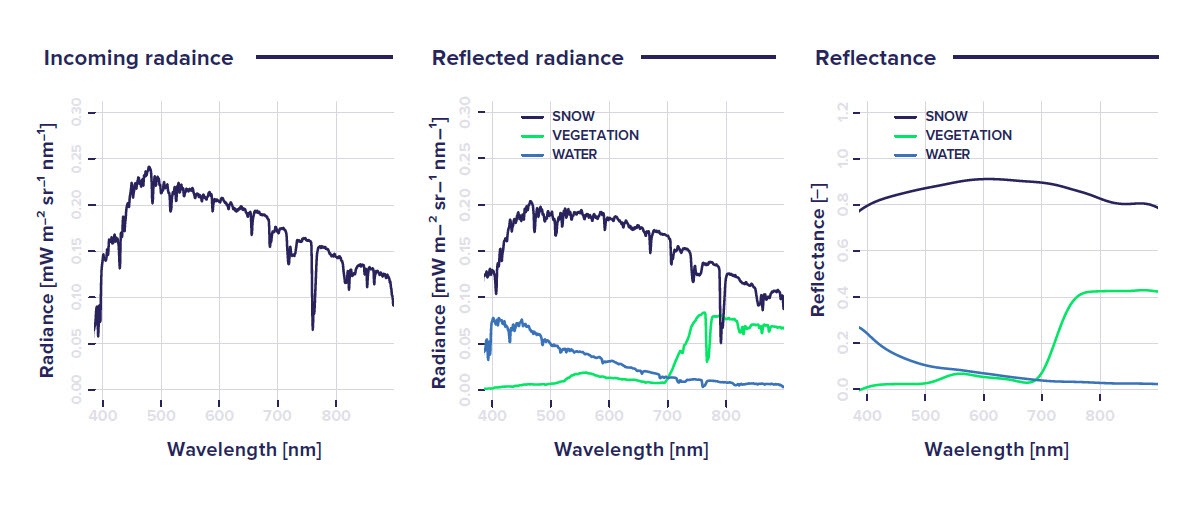